Using the analysis
module
The analysis
module contains a small selection of useful analysis tool that can be use to extract informations from GammaSpectrum
objects.
Searching peaks in a spectrum
The analysis
module provides a simple peak-search function based around the scipy.signal.find_peaks
function. The search is based on a user-specified prominence value.
A peak search can be easily performed giving the desired spectrum to the peak_search
function specifying a prominence value. The function returns a dictionay containing as key an integer index of the peak and as values a list containing the channel index corresponding to the peak maximum, its intensity in counts per seconds per channel and, if a calibration has been applied to the spectrum, the energy value of the maximum. As an example let us apply a peak search to the \(^{226}\text{Ra}\) presented in the previous sections.
Let us load the sample and background spectra together with the calibration file and let us generated a smoothed out difference spectrum to be used in the peak search.
from pygammaspec.spectrum import GammaSpectrum, Calibration
from pygammaspec.visualization import plot_spectrum
sample = GammaSpectrum.from_PRA_histogram("../utils/weak_radium.txt", 25851)
background = GammaSpectrum.from_PRA_histogram("../utils/background.txt", 25851)
calibration = Calibration.from_calibration_file("../utils/calibration.txt")
spectrum = (sample-background).average_smoothing(10)
spectrum.calibration = calibration
plot_spectrum(spectrum, enrange=(0, 800), yrange=(0, 0.0075))
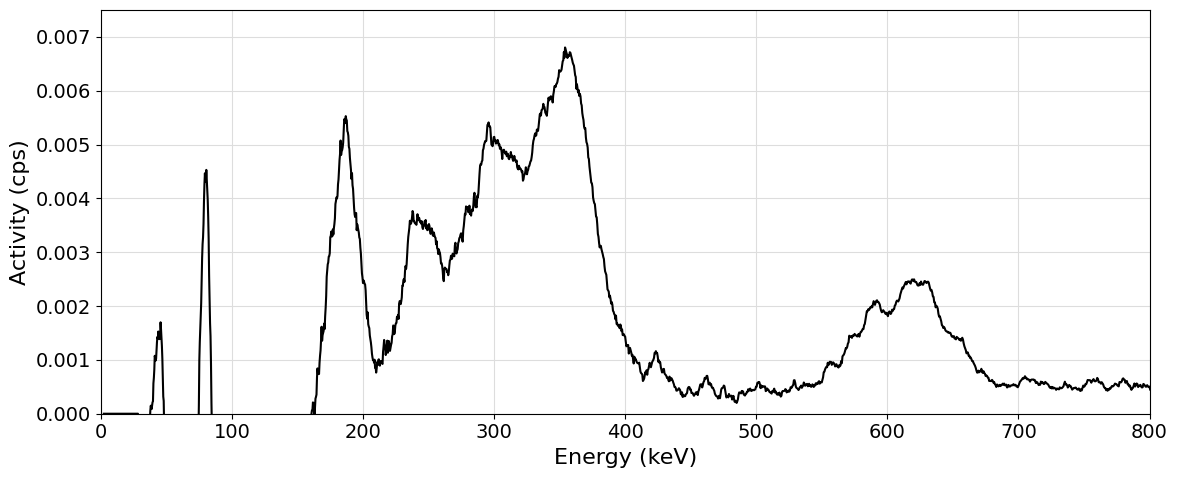
Now that the desired spectrum
has been obtained let us perform a peak search using the peak_search
function:
from pygammaspec.analysis import peak_search
peaks = peak_search(spectrum, prominence=0.001)
for i, (channel, counts, energy) in peaks.items():
print(f"{i}: {energy:.2f} keV \t{counts:.2e} cps\t\t(channel: {channel:.2f})")
0: 45.60 keV 1.70e-03 cps (channel: 1.40)
1: 80.50 keV 4.53e-03 cps (channel: 2.40)
2: 186.87 keV 5.53e-03 cps (channel: 5.23)
3: 237.85 keV 3.77e-03 cps (channel: 6.49)
4: 295.81 keV 5.41e-03 cps (channel: 7.86)
5: 354.15 keV 6.80e-03 cps (channel: 9.18)
6: 620.40 keV 2.50e-03 cps (channel: 14.62)
Please notice how the peak_search
function is also embedded into the plot_spectrum
function from the visualization
module. If a prominence value is specified in the call to plot_spectrum
, markers with either energy or channel values (depending on the plot mode) will be plotted on the spectra:
plot_spectrum(spectrum, enrange=(0, 800), yrange=(0, 0.009), prominence=0.001)
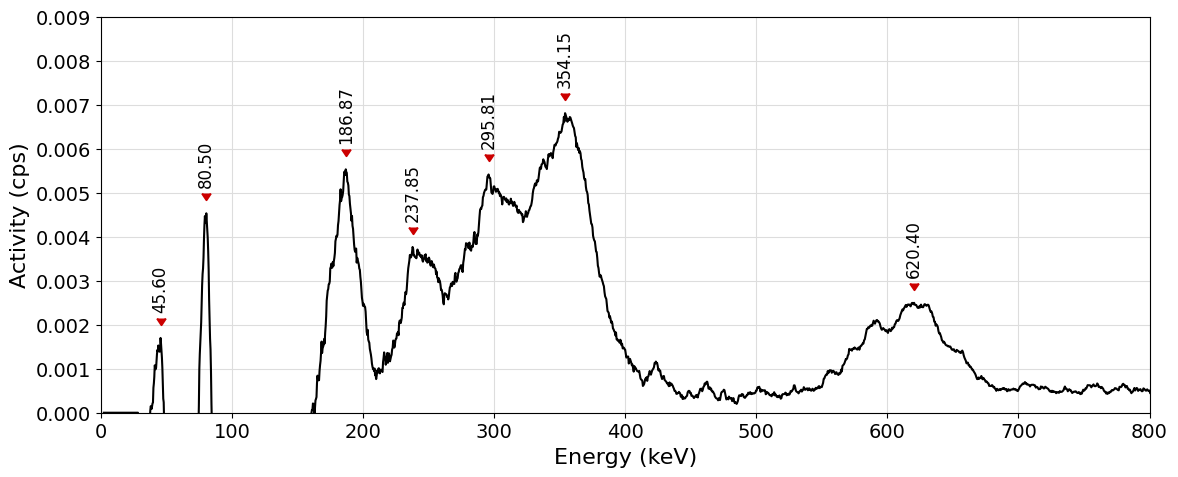
Fitting single peaks in the spectrum
The analysis
module provides a simple peak-fit function based around the scipy.optimize.curve_fit
function. The function can be used to fit peaks in a GammaSpectrum
object once it has been calibrated. Given a region of initerest, the alogrithm fits the experimental datapoints with a Gaussian function of unitary height corrected by a polinomial baseline of a user-defined order \(N\). The expression of the fitting function \(f(\varepsilon)\), written in terms of the vector \(\mathbf{c}=[c_0, c_1, c_{N+3}]\) of optimized parameters, is the following:
where \(\varepsilon\) represents the energy in \(\text{keV}\), \(c_0\) represents the height of the gaussian fitting the peak, \(c_1\) represents the energy in \(\text{keV}\) at which the peak is located, \(c_2\) represents the standard deviation of the Gaussian while the coefficients \(\{c_3, ..., c_{N+3}\}\) represents the coefficients of the polynomial expansion.
The peak_fit
function retuns many objects. In the order:
A
list
offloat
values of length \(N+4\) representing the vector \(\mathbf{c}\) of optimized parametersA
list
offloat
values representing the section of energy values used in the fitting procedure.A
list
offloat
values containing the function profile in the considered energy region.A
list
offloat
values containing the baseline profile in the considered energy region.A
float
value representing the estimated efficiency of the detector on the fitted peak.
Note
The efficiency \(\eta\) of the detector is computed based on the ratio of the full-width at half maximum (\(\text{FWHM}\)) of the gaussian fitting the peak and the energy \(\varepsilon_0\) at which the peak is centered.
or, in terms of the optimized coefficents:
To show how the peak fitting function can be used let us consider the peak of \(^{214}\text{Bi}\) at \(609.9 \text{keV}\) in the previously obtained spectrum. Let us perform a fitting in the region from \(475\text{keV}\) to \(750\text{keV}\) using a linear baseline (order 1):
from pygammaspec.analysis import fit_peak
popt, efit, yfit, bfit, efficiency = fit_peak(spectrum, 475, 800, baseline_order=1)
print("Fitting results:")
print("----------------------------------------")
print(f"Central energy: {popt[1]:.2f} keV")
print(f"Peak height: {popt[0]:.2e} cps")
print(f"Peak STD: {popt[2]:.2f} keV^2")
Fitting results:
----------------------------------------
Central energy: 614.02 keV
Peak height: 1.92e-03 cps
Peak STD: 32.14 keV^2
The fitting results can be easily plotted using the data provided by the fitting function:
import matplotlib.pyplot as plt
fig = plt.figure(figsize=(8, 6))
plt.plot(spectrum.energy, spectrum.counts, c="black", zorder=3)
plt.plot(efit, yfit, c="blue", zorder=3)
plt.plot(efit, bfit, c="red", zorder=4)
plt.fill_between(efit, yfit, bfit, color="#00AAAA", alpha=0.5, zorder=3)
plt.xlim((300, 900))
plt.ylim((0, 0.003))
plt.xlabel("Energy (keV)", size=14)
plt.ylabel("Counts (cps)", size=14)
plt.grid(which="major", c="#DDDDDD")
plt.tight_layout()
plt.show()
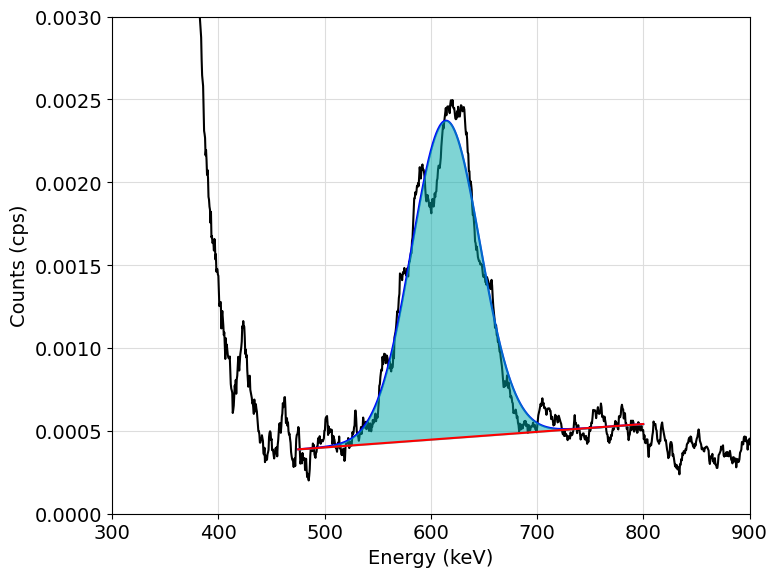